Release Notes - 0.8.0
Furiosa SDK 0.8.0은 메이저 릴리즈로 다수의 성능 개선, 기능 추가, 버그픽스를 포함한다. 0.8.0 릴리즈는 사용자 응용 개발의 핵심 도구인 서빙 프레임워크와 Model Zoo에 대한 주요한 개선을 포함한다.
패키지 이름 |
버전 |
---|---|
NPU Driver |
1.4.0 |
NPU Firmware Tools |
1.2.0 |
NPU Firmware Image |
1.2.0 |
HAL (Hardware Abstraction Layer) |
0.9.0 |
Furiosa Compiler |
0.8.0 |
Python SDK (furiosa-runtime, furiosa-server, furiosa-serving, furiosa-quantizer, ..) |
0.8.0 |
NPU Device Plugin |
0.10.1 |
NPU Feature Discovery |
0.2.0 |
NPU Management CLI (furiosactl) |
0.10.0 |
설치 또는 업그레이드 방법
APT 저장소를 사용하고 있다면 다음과 간단히 업그레이드할 수 있다.
apt-get update && apt-get upgrade
특정 패키지 지정해서 업그레이드 하고 싶다면 다음과 같이 실행 한다. APT 저장소 설정 및 설치 방법에 대한 자세한 설명은 드라이버, 펌웨어, 런타임 설치 가이드 에서 찾을 수 있다.
apt-get update && \ apt-get install -y furiosa-driver-pdma furiosa-libhal-warboy furiosa-libnux furiosactl
펌웨어 업그레이드는 다음과 같이 실행한다.
apt-get update && \ apt-get install -y furiosa-firmware-tools furiosa-firmware-image
파이썬 패키지 업그레이드는 다음과 같이 실행한다.
pip install --upgrade furiosa-sdk
주요 변경 사항
서빙 프레임워크 API 개선
furiosa-serving 은 FastAPI 기반의 서빙 프레임워크이다. furiosa-serving 을 이용하면 사용자는 FastAPI를 기반으로 NPU를 활용하는 Python 기반 고성능 웹 서비스 응용을 빠르게 개발할 수 있다. 0.8.0 릴리즈에서는 다음 주요 업데이트를 포함한다.
다수의 NPU를 이용하여 서빙할 수 있는 Session Pool 기능 추가
높은 성능을 요구하는 응용이라도 다수의 작은 요청으로 변환 가능하다면 Session Pool 기능을 이용하여 다수의 NPU를 장착하여 성능을 향상 시킬 수 있다.
model: NPUServeModel = synchronous(serve.model("nux"))(
"MNIST",
location="./assets/models/MNISTnet_uint8_quant_without_softmax.tflite",
# Specify multiple devices
npu_device="npu0pe0,npu0pe1,npu1pe0"
worker_num=4,
)
스레드 기반으로 동작하던 NPU에 대한 요청 처리 방법을 asyncio 기반으로 개선
따라서 작고 빈번한 NPU 추론 요청도 더 낮은 지연시간으로 처리할 수 있다. 다음 예제와 같이 한번의 API 요청에 의해 다수의 NPU 추론을 처리하는 응용도 더 고성능으로 처리할 수 있게 되었다.
async def inference(self, tensors: List[np.ndarray]) -> List[np.ndarray]:
# The following code runs multiple inferences at the same time and wait until all requests are completed.
return await asyncio.gather(*(self.model.predict(tensor) for tensor in tensors))
다른 장치 및 외부 런타임 지원 확장 추가
복잡한 서빙 시나리오에서는 NPU를 기반으로 하는 Furiosa Runtime 외에도 다른 장치와 다른 런타임 프로그램을 사용할 필요가 있다. 이번 0.8.0 릴리즈에서는 다른 장치와 런타임을 사용할 수 있도록 프레임워크를 확장했으며 첫번째 외부 런타임으로 OpenVINO를 추가하였다.
imagenet: ServeModel = synchronous(serve.model("openvino"))(
'imagenet',
location='./examples/assets/models/image_classification.onnx'
)
S3 클라우드 스토리지 저장소 지원
Model의 location
에 S3 URL을 지정하면 된다.
# Load model from S3 (Auth environment variable for aioboto library required)
densenet: ServeModel = synchronous(serve.model("nux"))(
'imagenet',
location='s3://furiosa/models/93d63f654f0f192cc4ff5691be60fb9379e9d7fd'
)
OpenTelemetry 호환 트레이싱 지원
OpenTelemetry Collector 기능을 이용하여 서빙 어플리케이션의 특정 코드 구간의 실행 시간을 추적할 수 있는 기능이 추가되었다.
이 기능 활용을 위해서는 아래와 같이 trace.get_tracer()
호출해 tracer를 초기화 하고
tracer.start_as_current_span()
함수를 호출하여 구간을 지정해주면 된다.
from opentelemetry import trace
tracer = trace.get_tracer(__name__)
class Application:
async def process(self, image: Image.Image) -> int:
with tracer.start_as_current_span("preprocess"):
input_tensors = self.preprocess(image)
with tracer.start_as_current_span("inference"):
output_tensors = await self.inference(input_tensors)
with tracer.start_as_current_span("postprocess"):
return self.postprocess(output_tensors)
그리고 OpenTelemetry Collector 의 지정은
다음과 같이 FURIOSA_SERVING_OTLP_ENDPOINT
환경설정을
통해 할 수 있다. 다음 그림은 Grafana를 통해 트레이싱 결과를 시각화한 결과의 예이다.
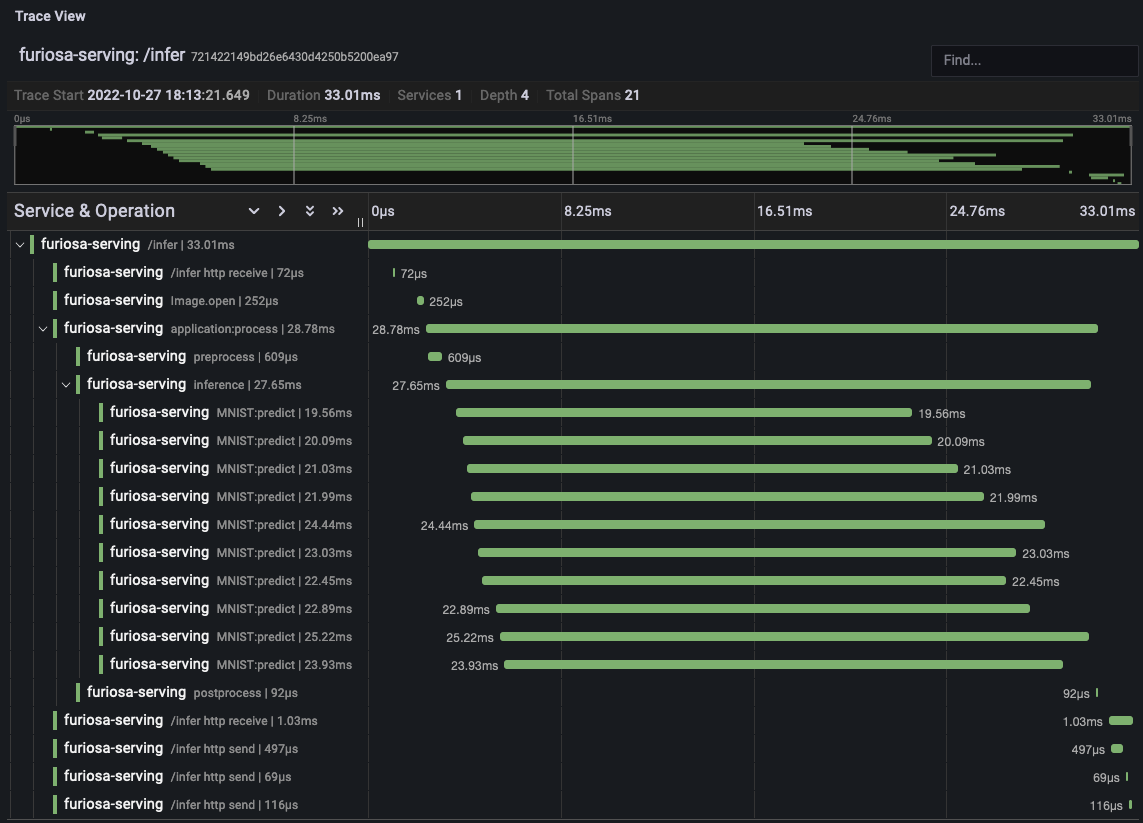
그 외의 다른 중요한 개선점은 다음과 같다:
컴파일러 설정
batch_size
를 서빙 API에서 지원하여 다수 입력 샘플을 한번에 실행할 수 있도록 개선Session 옵션
worker_num
을 서빙 API에서 지원하여 더 많은 스레드가 NPU를 공유할 수 있도록 개선
프로파일러 개선
프로파일러 트레이싱 결과를 데이터 분석 프레임워크인 Pandas 를 이용해 분석할 수 있는 기능이 추가되었다. 이 기능을 이용하면 트레이싱한 결과 데이터를 분석하여 병목지점 식별 및 모델성능 원인을 빠르게 파악할 수 있다. 자세한 사용법은 Pandas DataFrame을 이용한 트레이스 분석 에서 찾을 수 있다.
from furiosa.runtime import session, tensor
from furiosa.runtime.profiler import RecordFormat, profile
with profile(format=RecordFormat.PandasDataFrame) as profiler:
with session.create("MNISTnet_uint8_quant_without_softmax.tflite") as sess:
input_shape = sess.input(0)
with profiler.record("record") as record:
for _ in range(0, 2):
sess.run(tensor.rand(input_shape))
df = profiler.get_pandas_dataframe()
print(df[df["name"] == "trace"][["trace_id", "name", "thread.id", "dur"]])
모델 양자화 도구 (quantization tool) 관련 개선
모델 양자화 는 이미 트레이닝 된 모델을 양자화된 모델로 변환해주는 도구이다. 0.8.0 릴리즈는 다음 주요 개선 사항을 포함하고 있다.
SiLU 연산자 처리 정확도 향상
컴파일러 설정
without_quantize
을 사용하기 쉽도록 개선MatMul/Gemm 연산자 처리 정확도 향상
Add/Sub/Mul/Div 연산자 처리 정확도 향상
Conv/ConvTranspose/MaxPool 연산자 처리가 더 다양한 auto_pad 속성에 대해서도 NPU 가속될 수 있도록 개선
PRelu 연산자가 NPU 가속 가능하도록 개선
furiosa-toolkit 개선
furiosa-toolkit 0.10.0 릴리즈에 포함된 furiosactl
명령행 도구는
다음과 주요 개선 내용을 포함한다.
새로 도입된 furiosactl ps 명령은 어떤 OS 프로세스가 NPU 장치를 점유하고 있는지 출력한다.
# furiosactl ps
+-----------+--------+------------------------------------------------------------+
| NPU | PID | CMD |
+-----------+--------+------------------------------------------------------------+
| npu0pe0-1 | 132529 | /usr/bin/python3 /usr/local/bin/uvicorn image_classify:app |
+-----------+--------+------------------------------------------------------------+
furiosactl info 명령은 각 장치 고유의 UUID를 출력하도록 개선 되었다.
$ furiosactl info
+------+--------+--------------------------------------+-----------------+-------+--------+--------------+---------+
| NPU | Name | UUID | Firmware | Temp. | Power | PCI-BDF | PCI-DEV |
+------+--------+--------------------------------------+-----------------+-------+--------+--------------+---------+
| npu0 | warboy | 72212674-61BE-4FCA-A2C9-555E4EE67AB5 | v1.1.0, 12180b0 | 49°C | 3.12 W | 0000:24:00.0 | 235:0 |
+------+--------+--------------------------------------+-----------------+-------+--------+--------------+---------+
| npu1 | warboy | DF80FB54-8190-44BC-B9FB-664FA36C754A | v1.1.0, 12180b0 | 54°C | 2.53 W | 0000:6d:00.0 | 511:0 |
+------+--------+--------------------------------------+-----------------+-------+--------+--------------+---------+
furiosactl 의 설치 및 사용법에 대한 자세한 내용은 furiosa-toolkit 에서 찾을 수 있다.
Model Zoo API 개선, 모델 추가, 네이티브 후처리 코드 추가
furioa-models 는 FuriosaAI NPU에 최적화된 모델을 제공하는 공개 Model Zoo 프로젝트이다. 0.8.0 릴리즈는 다음 주요 개선 사항을 포함한다.
YOLOv5 Large/Medium 모델 추가
SOTA 객체탐지(Object Detection) 모델인 YOLOv5l
, YOLOv5m
가 추가되었다.
사용 가능한 전체 모델은
모델 리스트 에서 찾아볼 수 있다.
모델 클래스 및 로딩 API 개선
모델 클래스가 개선되어 전후처리 코드를 포함하고 모델 로딩 API는 다음과 같이 개선되었다. 그 외 모델 클래스와 API에 대한 자세한 내용은 Model Object 에서 찾아볼 수 있다.
기존 코드
from furiosa.models.vision import MLCommonsResNet50
resnet50 = MLCommonsResNet50()
변경된 코드
from furiosa.models.vision import ResNet50
resnet50 = ResNet50.load()
기존 코드
import asyncio
from furiosa.models.nonblocking.vision import MLCommonsResNet50
resnet50: Model = asyncio.run(MLCommonsResNet50())
0.8.0 개선 사항
import asyncio
from furiosa.models.vision import ResNet50
resnet50: Model = asyncio.run(ResNet50.load_async())
모델의 후처리는 추론 출력인 텐서를 어플리케이션에서 접근하기 좋은 구조적 데이터로 변경해주는 과정으로 모델에 따라 긴 실행 시간을 차지할 수 있다. 0.8.0 릴리즈는 ResNet50, SSD-MobileNet, SSD-ResNet34를 위한 네이티브 후처리 코드를 포함했으며 자체 성능 평가에 의하면 네이티브 후처리 코드는 모델에 따라 최대 70%까지 지연시간(latency)을 격감 시킬 수 있다.
다음은 ResNet50의 네이티브 후처리 코드를 사용하는 전체 예제이다. 더 자세한 내용은 Pre/Postprocessing 에서 찾아 볼 수 있다.
from furiosa.models.vision import ResNet50 from furiosa.models.vision.resnet50 import NativePostProcessor, preprocess from furiosa.runtime import session model = ResNet50.load() postprocessor = NativePostProcessor(model) with session.create(model) as sess: image = preprocess("tests/assets/cat.jpg") output = sess.run(image).numpy() postprocessor.eval(output)
그 외의 변경 사항은 Furiosa Model - 0.8.0 Changelogs 에서 찾아볼 수 있다.